Artificial intelligence (AI) has rapidly transformed industries worldwide. From healthcare to entertainment, AI’s influence is undeniable. Yet, despite its remarkable achievements, the AI field faces significant challenges today. Issues like data saturation and hardware limitations are slowing progress in large language models (LLMs). These barriers raise crucial questions about the future of AI.
The Current State of AI Innovation
Recent advancements in AI, such as ChatGPT and Google Bard, showcase the power of LLMs. These tools are trained on massive datasets, enabling them to generate human-like responses. However, this progress is hitting a wall. The saturation of high-quality data and computational hardware bottlenecks are limiting further development. According to The Times, researchers are struggling to find new data to train models effectively.
Why Data Saturation Is a Problem
AI models require diverse and extensive datasets for training. However, much of the high-quality data has already been utilized. This saturation makes it harder to improve AI performance without resorting to lower-quality or redundant data. Re-training models with subpar datasets often leads to diminishing returns. As The Times notes, companies like OpenAI are exploring alternative strategies, such as synthetic data generation, to address this issue.
The Hardware Bottleneck
AI models also demand enormous computational resources. GPUs (graphics processing units) are essential for training and deploying LLMs. However, the current hardware infrastructure struggles to meet the rising demand. NVIDIA, a leading GPU manufacturer, has reported shortages due to the increasing workload required for AI projects. This scarcity has slowed down innovation in AI development.
Furthermore, power consumption is a growing concern. Large-scale AI training consumes vast amounts of energy, contributing to environmental challenges. Addressing these bottlenecks will require not only better hardware but also more sustainable practices. Barron’s highlights efforts by tech giants to develop energy-efficient AI chips.
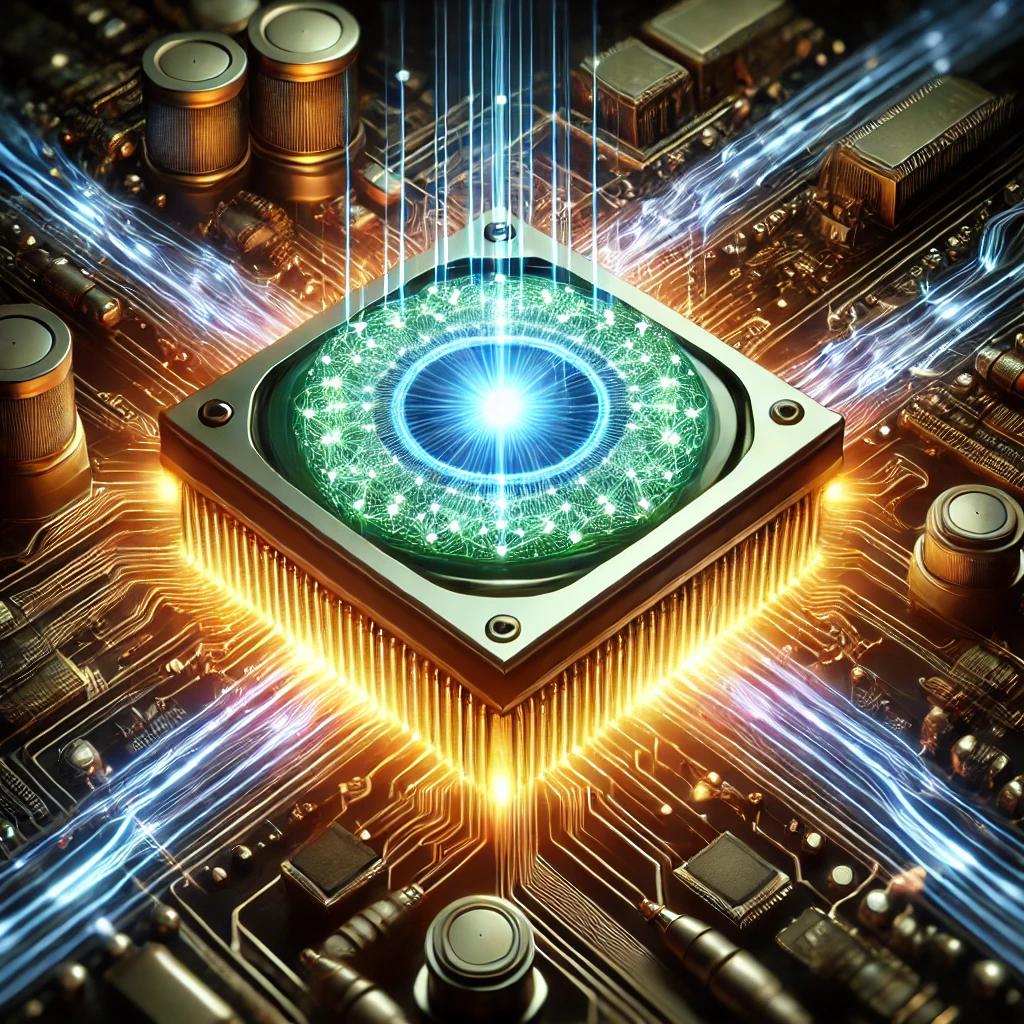
Potential Solutions on the Horizon
Several companies are investing in new approaches to overcome these limitations. For example:
- Custom Hardware: NVIDIA and Google are developing specialized chips designed for AI workloads. These chips aim to reduce power consumption while enhancing performance.
- Synthetic Data: Researchers are creating artificial datasets to train AI models without relying on real-world data. Synthetic data can help bridge the gap caused by data saturation.
- Collaborative Efforts: Companies are collaborating to share resources and advance AI research. Initiatives like OpenAI’s partnerships foster innovation despite challenges.
What This Means for the Future
Despite these challenges, the future of AI remains promising. Addressing data and hardware limitations will unlock new possibilities. Industries can expect smarter applications, better efficiency, and wider adoption of AI-driven solutions.
As these solutions evolve, the AI landscape will continue to transform. Collaboration between tech companies, researchers, and policymakers will be critical. Together, they can overcome the obstacles holding back AI innovation.
Final Thoughts
AI has already revolutionized the world, but its journey is far from over. By addressing the current challenges of data saturation and hardware constraints, the industry can ensure sustainable growth. With innovative solutions on the horizon, the future of AI remains as exciting as ever.
For a deeper dive into these challenges, explore insights from The Times, Barron’s, and other industry experts. The journey ahead is complex, but it’s filled with potential for groundbreaking innovations.
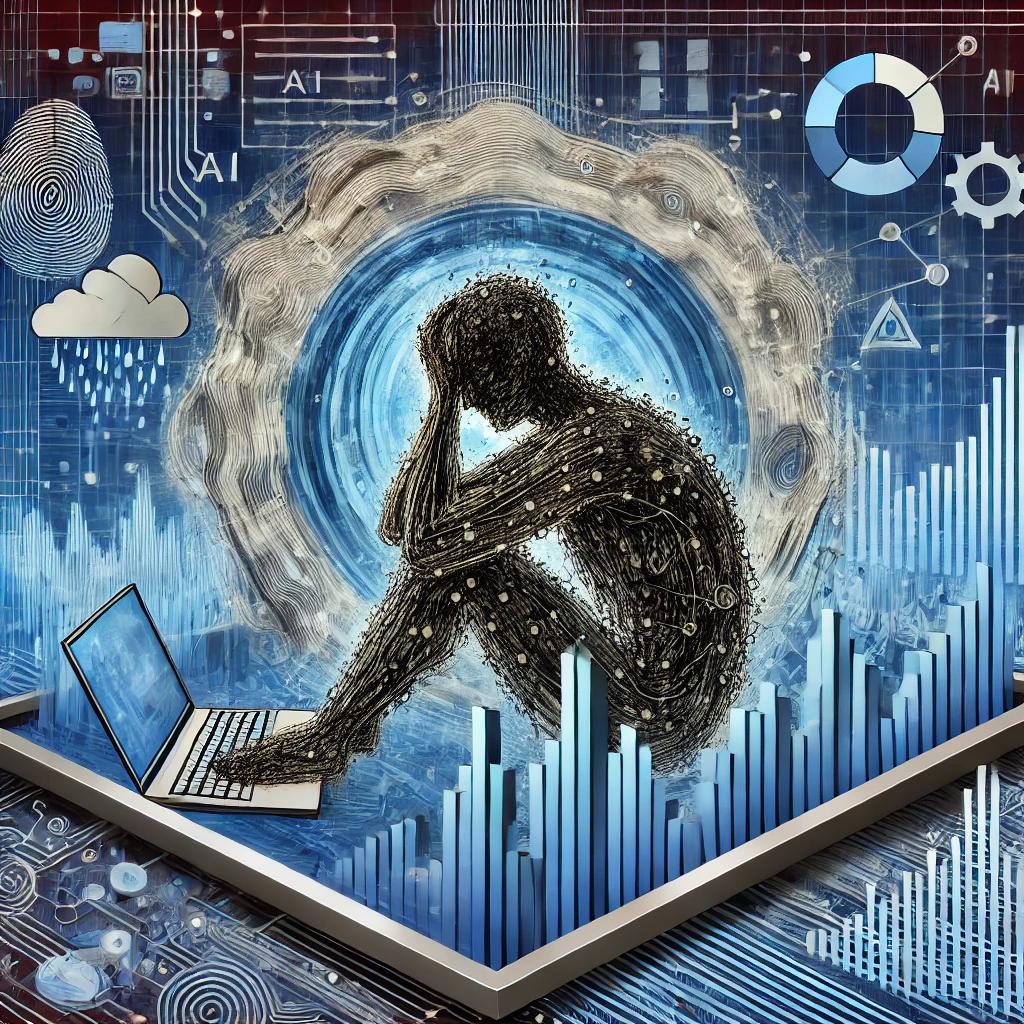